Sigma Q&A Part II: Does Foveon’s Quattro sensor really out-resolve conventional 36-megapixel chips?
posted Tuesday, April 8, 2014 at 2:24 PM EST
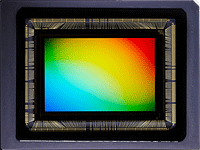
Sigma Corporation's image sensor subsidiary, Foveon Inc., takes a very different approach to sensor design than any other company out there. With a technology that captures red, green, and blue light simultaneously at all pixel locations across the entire sensor surface, they've long claimed that their chips out-resolve conventional Bayer-array imagers on a per-pixel basis by a significant margin.
Their most recent sensor tech, dubbed Quattro, takes another new turn. With green and red layers that have a lower resolution than the topmost blue layer, the company still claims a much higher "equivalent" resolution than the number of pixels (or more properly, photodiodes) on the chip would indicate. The new Quattro chip has 19.6 million photodiodes on its topmost layer, but Foveon claims resolution equivalent to a 39-megapixel conventional chip.
Can this really be true, or is it just another example of, shall we say, "optimistic" marketing?
While at the CP+ show in Yokohama, Japan this past February, I sat down with Sigma Corporation's CEO Kazuto Yamaki, along with Foveon's General Manager Shri Ramaswami and Vice President of Applications, Rudy Guttosch. We published the first part of that interview -- primarily a conversation with Mr. Yamaki -- last week. In this second part, I delve into the details of how the Quattro technology works, and look at the aggressive resolution claims.
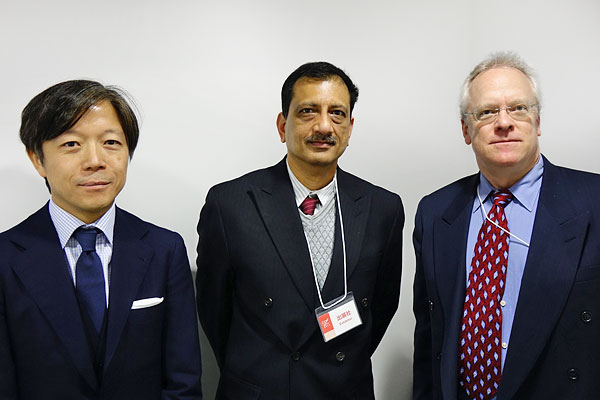
Some background: Foveon's X3 sensor technology vs. conventional approaches
Before plunging into the interview itself, it would help to understand a bit about Foveon's sensor tech, and what makes it so different than anything else on the market. Our discussion here will necessarily be limited, but here are some resources for people who want to dig a little deeper: Foveon's page explaining X3 technology, another explanation courtesy of Wikipedia, and for those uber-geeks who really want to get down in the weeds, a Foveon white paper on X3 tech.
Most conventional image sensors use an array of color filters spread across the surface of the chip to separate light into red, green, and blue components, such that each individual photodiode sees only red, green, or blue light. The most common arrangement of color filters is the so-called Bayer filter, developed by Bryce Bayer in 1974 while working for Eastman Kodak Corporation. The Bayer color filter array is akin to a checkerboard pattern, and has twice as many green-sensitive photodiodes as it does red or blue.
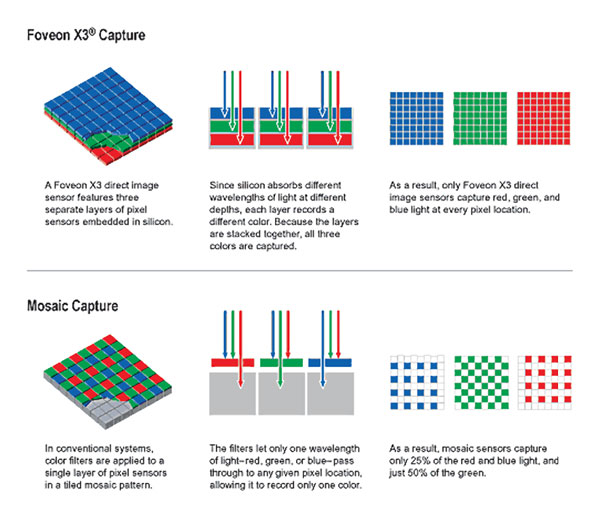
By comparison, Foveon's X3 sensor technology takes advantage of how silicon absorbs light, and determines color information based on how far the light penetrates beneath the surface of the silicon. As a result every pixel has complete red, green and blue data available.
The image above illustrates this, comparing a Bayer-filtered sensor with a Foveon X3 chip. Looking at the two different approaches, it seems reasonable that the Foveon array would have higher resolution than a conventional "striped" or mosaic array with the same number of pixels. After all, the mosaic approach somehow has to combine the separate red, green, and blue data into the full-color pixels that the Foveon sensor starts out with. The mosaic array is quite simply capturing less information.
The question, of course, is just how much more resolution a Foveon chip has than a conventional one, and how to characterize it. The short answer, at least according to Foveon, is that their pixels produce about the same resolution as twice as many pixels on a mosaic sensor. To a first order of approximation, this somewhat makes sense, since the luminance (brightness or detail) channel in the human eye is closely correlated with the green channel. Since only half of the photodides a Bayer-filtered array are green-sensitive, it seems at least plausible that it would have about half the resolution of a sensor that provided green color data at every location.
We'll get into whether this is in fact true or not as we get into the interview itself, but first, there's another wrinkle: Foveon's new Quattro technology.
Foveon's Quattro technology: Fewer photodiodes, yet still the same resolution?
One of the limitations of Foveon's approach is that image noise is higher than in conventional sensors. This is probably due in part to inefficiencies within the sensor architecture itself -- perhaps some light is lost to internal structures that separate the layers -- and also in part to the processing that has to be done to produce pure colors from the rather mixed signals that the chip actually captures. (More on this momentarily in the interview.)
All else being equal, it's well known that larger photodiodes have better signal-to-noise ratios than smaller ones, but of course bigger photodiodes mean less resolution ... or do they? With the Quattro sensor design, Foveon appears to be trying to have its cake (high resolution; no color aliasing) and eat it too (by getting lower noise thanks to the larger photodiodes). Let's take a look at the Quattro architecture.
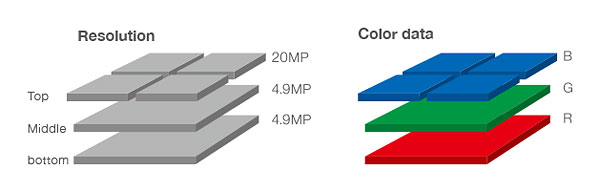
The illustration above shows the new Quattro sensor design. The top, blue layer has four times as many pixels as the lower green and red layers. Foveon claims that this arrangement produces the same overall resolution -- including the color resolution -- as does the X3 design described previously, while color noise is significantly reduced. That's an extraordinary claim, and one that seems counterintuitive as well, given that there are significantly fewer photodiodes for two out of the three layers.
This is what drove my interview with the Foveon execs at CP+. With the background out of the way, here's the interview itself.
Dave Etchells/Imaging Resource: We're kind of struggling at Imaging Resource with how to characterize the resolution of the Quattro sensor. It's described as having 39 effective megapixels, but it has the RGB photodiodes stacked, and also has different resolution on the green and red layers than the blue one. Of course, the luminance resolution of a conventional Bayer-striped sensor is something less than the total pixel count as well. How did you calculate that 39 megapixel number?
Shri Ramaswami/Foveon: As you mentioned, whether we're talking about a Bayer sensor or something like a Foveon X3, the relationship between what is on the sensor and what is in the output is not such a simple matter. In this case, though, the resolution -- just like in the Merrill [cameras' sensors] -- is fairly simple. In other words, you look at the highest resolution channel that we have: In this case, we call it the top layer. As you'd said, we compare it against the luminance resolution of a Bayer pattern. It comes up to a factor of two.
DE: It's pretty-much just a straight factor of two?
SR: Pretty much. It comes out exactly, generation after generation, you just hit it.
DE: And that's based on direct measurements with Bayer sensors?
SR: And of course, we've measured it. It comes out of the math, it has to be that way, and we make sure that the results hold up to the math. That's really what we're after -- get that higher resolution and keep it consistent among the colors. All our measurements are indicating that, and have shown it consistently.
DE: That's a valid number, then -- the math supports the measurements, and measurements support the math.
SR: Yes, the measurements support the math.
DE: So in a Bayer-striped sensor, basically, luminance follows the green channel in our eyes, and there's only half as many green pixels, so you say a 20-megapixel sensor is only 10 megapixels in luminance resolution. But you get some luminance information from the red and blue too, in the processing.
SR: You do. Of course, as you just said, luminance is not just pure green; but in reality, the green color filter characteristic follows what we call the human eye response very, very nicely in terms of perception. So, the majority of it comes from there. The other reason that it comes in there is that the sampling of red and blue is so much smaller. [Ed. Note: Meaning lower resolution.]
The real contribution becomes the matter of, what is the type of object that you have? It depends very much on the subject matter that you have. You can get closer to what you should get out of a full sensor, but never quite. In some cases, as you know, like in moiré situations, it breaks down quite badly -- and we see that because the colors are not resolved the same way. Which is why you get color moiré, it's that simple. It can break pretty badly, or it can be, in large regions of color; doesn't really matter how you sample it. It has much more to do with, you know, the smooth tonality that you get with higher resolution.
DE: Yeah, that's interesting. In one of our test targets I made some little rosettes, so there's red, green, and blue with black wedges, and then we have red against green and blue against green. It's interesting, you can really see the big difference. The green against black is the sharpest, cameras resolve furthest in the center. But especially red against blue, it's much, much softer. Whereas the Foveon sensors, they're all pretty equal.
SR: Yeah, that's something we check for -- resolution that's pretty consistent.
DE: When Kazuto and I were talking earlier during my headquarters visit, he said that the top channel of the Foveon chip, you began to call it "blue channel" because that's where all of the blues were; but it's also absorbing other wavelengths, too, so it's really some kind of a luminance channel. Can you talk a little bit about that to our readers? Maybe I just said everything that you'd say anyway, but how would you explain how you extract the luminance to get that resolution?
SR: As you said, when you absorb [light] into silicon, which is our fundamental principle of separating color information, you don't get the clean "this is blue"; in fact, all three channels absorb all wavelengths, to a certain extent.
Kazuto Yamaki/Sigma Corporation: We can share the spectral response.
SR: Oh yes, of course.
DE: A spectral curve? Oh yes, that'd be great.
SR: We can fire that up, and meanwhile I can talk. So the point is that in reality -- and you get a lot of broad overlap -- similar to the way the human eye actually responds, the way the retina responds to light, and so because of this broad characteristic, you have a lot of leeway in how you extract information. All three channels in reality are recording color and recording detail. Now it's a matter of, which is the most technologically simple way to do it? Which is the lowest-noise way to extract the information?
DE: Right, right. And of course, the deepest channel, the red channel has very little blue in it at that point -- it's fairly far down.
SR: It doesn't have a lot of it.
DE: But there's actually still a little bit.
SR: There is still some, and of course it has quite a lot of green information in it.
DE: Yeah.
SR: And of course, there are some disadvantages with this approach. Everyone knows our high ISO, for instance, isn't up to the standards of the competition.
DE: Yes, you're taking differences of numbers to extract the color, so the noise is somewhat magnified.
SR: Yes, it's a subtractive color system, but the advantage of that is that you can stack them. If they were too narrow, you couldn't stack them. That brings super-high clarity to it.
DE: The Quattro sensor is different because it has lower resolution in the red and green channels. That makes sense, because it helps you keep the noise down thanks to a larger photodiode area. Can you describe anything about how the camera's processor goes about separating luminance and colors?
SR: We have that slide, too, in our presentation. Yes. It's a simplified version of it, but it basically goes back to the fundamental difference between what we have been calling it. It was a simplified way of saying red, green and blue. As you know, it really isn't. None of them are a pure color, so in reality, what it allows you do to -- to separate out the detail information, but also be able to get the color out, and the detail, or the color out of the rest... this is our special characteristic. So, you know, the top, middle and bottom layers. [Ed. Note: The blue curve corresponds to the top layer, the green curve to the middle one, and the red curve to the bottom layer of the sensor.]
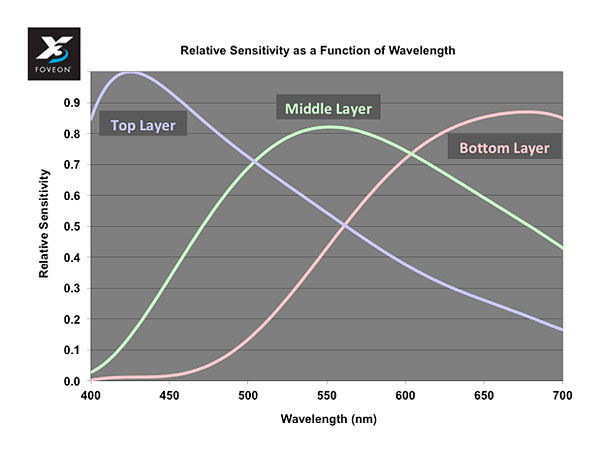
SR: You can see that the top layer is blue-heavy, but it's not blue. The next layer is green-heavy, but it isn't green. The bottom is red-heavy. None of these is just red, green, or blue -- that really allows you to do something very interesting. So this is fundamentally a pretty smart way to keep your information, but at the same time, reduce the whole load on the system, because these things are not pure colors. It may sound counterintuitive, but it actually allows you to separate out very cleanly all the detail information -- as we call it -- from the top layer, and understand where the color detail comes from. In other words, it allows us to actually get back what was apparently lost.
DE: I see. Because each layer has all the colors, you can correlate. So you can look at the top layer and say "Okay, we know we've got some red here," then you look at the red layer to see how much is there, and you can sort of take that out.
SR: Precisely -- it creates the correlation automatically, and therefore, you can remove some redundant information that you didn't actually need. Part of the advantage that you get from that is that you are then able to increase the signal.
DE: That's an interesting concept. You're taking advantage of redundancy in the signal to simplify the processing, and also help with the noise levels.
SR: Which is why the spectral response is so important -- because it tells you there is actually more information than just the spatial, three-dimensional structure to work from. If you utilize that additional information...
DE: Yeah, it's not like you just have a blue filter on top of everything.
SR: It would be impossible then, without that correlation, to do this.
DE: That's very, very interesting. There has to be some kind of trade-off -- nothing comes for free. Is there likely to be any less resolution in the green and red at all? I wouldn't think you would have color aliasing, but I wonder what the consequence would be?
SR: This is another thing that Kazuto-san is showing this afternoon, but here for example is a 36-megapixel Bayer pattern image, and this is Quattro... [showing me the image below]
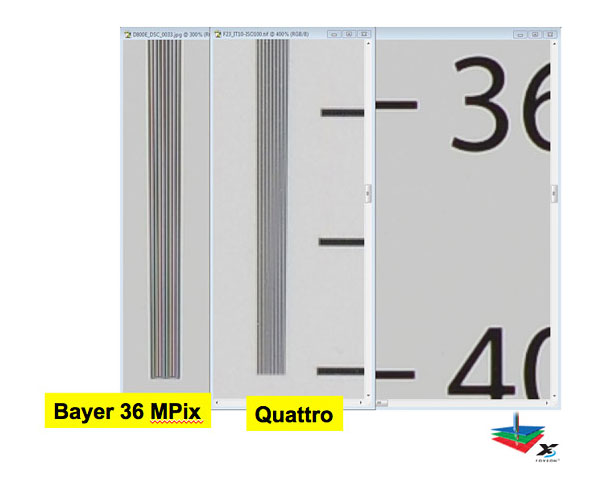
(Note: Images are courtesy of Foveon, and are not Imaging Resource test shots.)
DE: Wow, very interesting.
SR: Quattro is very clean, so we're able to maintain that, you know... This is really key for us, right. We examine things to make sure that things stay clean, the detail.
DE: That signals all of them that there is just black and white.
SR: This is not a black and white; the image, as you can see...
DE: Well, yeah, it's a color image, but the subject is black and white.
SR: Yes, the subject is black and white, which is why it becomes so obvious when the rendering is no longer in black and white.
DE: Yes, you see the color aliasing with the Bayer. You don't see any color aliasing at all with the Quattro.
SR: You see luminance aliasing, for sure, that has to happen.
DE: Yes, of course it's going to alias in the luminance, but there's no color there at all.
SR: Our resolution is right where we expect it to be, and again, we can show you what it looks like against the Merrill [sensor] -- so you can see Merrill here. [showing me the res-chart crops below]
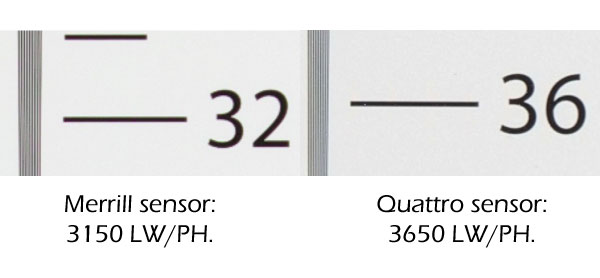
(Note: Images are courtesy of Foveon, and are not Imaging Resource test shots.)
SR: This is where it's supposed to end up, and this is Quattro. It's ending up where it's supposed to. Both of them are equally clean, and these are both color images, not black and white. Not throwing anything away.
DE: Very, very interesting.
SR: So, it may sound a little counterintuitive at the very beginning, but we went through it carefully to convince ourselves that it is really...
DE: It really is 39 megapixels, and you really don't have artifacts. You've separated the color and luminance.
SR: You don't have artifacts.
DE: Presumably, one of the advantages of going to larger green and red pixels is reduced image noise. Do you have a spec for how much ISO noise has been improved in the Quattro chip?
KY: Approximately one stop.
DE: About a full stop?
KY: Of course, it depends on the situation and the conditions...
DE: Yeah.
KY: ...but the average is one stop.
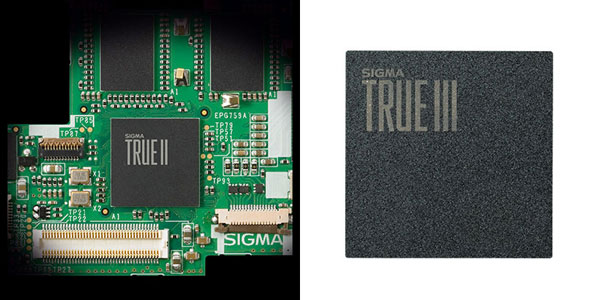
DE: And despite the higher pixel count -- and presumably more complicated image processing associated with all the sorting out of luminance and chrominance -- I believe when we were talking earlier, you said that the dp2 Quattro has the same two-to-three second-per-image processing?
KY: We used a newer processor.
DE: So it's a much more powerful processor. I think you said you had three processors and four analog front-ends?
Rudy Guttosch/Foveon: It's a True III processor that Kazuto-san was referring to, so that's the version number. There's one processor, and then four analog front-ends.
KY: One processor.
DE: Ah, one processor, I see -- but it's a more powerful processor.
Well, believe it or not, that was actually all of my questions. The charts and graphs are very helpful, and it'll be very interesting to get our hands on the dp2 Quattro. In the lab we have a Nikon D800E that we use for lens testing, so if we get the dp2 Quattro in, we can actually demonstrate this ourselves with our own targets. Thank you all very much for your time!